
Have you ever wondered what separates thriving businesses from those that struggle to grow?
How do some companies consistently make smarter decisions, predict customer needs, and increase revenue—while others seem to miss the mark? The answer often lies in one powerful tool: big data analysis.
Imagine being able to foresee market trends, optimize your pricing, or pinpoint exactly what your customers want.
But the real question is: how can your business leverage this data to increase profits? In this article, we’ll explore how big data analysis provides a competitive edge to help your company boost its revenue.
What is Big Data Analysis?
Big data analysis examines large, complex datasets to uncover hidden patterns, correlations, and other valuable insights.
It involves using advanced analytics techniques, such as machine learning, data mining, and predictive modeling, to make sense of the data and extract actionable intelligence.
Big data analysis aims to help businesses:
- Make data-driven decisions
- Optimize processes
- Identify new opportunities for growth
By leveraging the power of big data, companies can gain a deeper understanding of their customers, market trends, and operational performance.
Examples of Big Data Analysis in Business
Big data analysis has applications across various business functions and industries. Here are some examples:
- Analyzing customer behavior and preferences: By examining data from social media, website interactions, and purchase history, businesses can gain insights into customer preferences, segment their audience, and personalize marketing efforts.
- Optimizing supply chain and logistics: Big data analysis can help companies streamline their supply chain by identifying inefficiencies, predicting demand, and optimizing inventory management.
- Detecting and preventing fraud: Financial institutions and insurance companies use big data analysis to identify suspicious patterns and prevent fraudulent activities.
- Improving marketing campaigns and personalization: By analyzing customer data, businesses can create targeted marketing campaigns, recommend relevant products or services, and enhance the overall customer experience.
Benefits of Big Data Analysis for Businesses
Now that you understand what big data analysis is and how it works, let's explore the tangible benefits it offers to businesses.
1. Increased Revenue
One of the primary advantages of big data analysis is its potential to drive revenue growth.
By examining vast amounts of data, you can uncover new business opportunities and revenue streams that may have otherwise gone unnoticed.
For example, organizations that leverage big data report an average 8% increase in revenues and a 10% reduction in expenses.
Big data analysis also enables you to optimize pricing and promotions. You can adjust real-time prices to maximize profitability by understanding customer behavior and market trends.
Additionally, analyzing the effectiveness of past promotions can help you create more targeted and successful campaigns in the future.
2. Improved Customer Experience
Big data analysis empowers you to personalize products, services, and marketing efforts based on customer preferences and behaviors. In fact, 76% of consumers are more likely to choose a brand that offers personalized experiences.
By leveraging data from various touchpoints, such as website interactions, social media, and customer support channels, you can gain a 360-degree view of your customers and tailor your offerings accordingly.
Moreover, big data analysis can help you predict and prevent customer churn.
By identifying patterns and risk factors associated with customer attrition, you can proactively reach out to at-risk customers and offer personalized incentives to retain their business.
3. Enhanced Operational Efficiency
Big data analysis significantly improves operational efficiency by streamlining processes and reducing costs.
For instance, companies utilizing big data through AI-enabled supply-chain management can improve logistic costs by up to 15%.
For example, predictive maintenance powered by big data reduces equipment downtime in manufacturing.
Manufacturers prevent unexpected breakdowns by analyzing machine data and predicting when maintenance will be required, saving time and money.
General Electric, for example, uses big data to monitor its engines, ensuring they run optimally, saving millions in maintenance costs.
4. Better Decision Making
Big data analysis empowers you to make informed, data-driven decisions based on real-time insights.
Approximately 69% of organizations report that big data initiatives lead to better strategic decision-making, enabling them to respond more effectively to market changes.
You can comprehensively understand your business performance, customer behavior, and market trends by analyzing vast amounts of data from various sources. This enables you to make strategic decisions quickly and confidently rather than relying on intuition or limited information.
Furthermore, big data analysis helps you identify and mitigate potential risks. By detecting anomalies, fraud, or other threats early on, you can take proactive measures to protect your business and minimize the impact of adverse events.
5. Risk Management and Fraud Detection
Businesses can detect anomalies and flag potential fraud in real time by continuously analyzing transactions, especially in sectors like finance and e-commerce.
For example, banks use big data to monitor transactions and identify patterns indicative of credit card fraud.
This proactive risk management approach saves the institution and its customers from financial losses.
Risk management also includes anticipating operational and market risks, allowing companies to create contingency plans.
For example, airlines use big data to predict weather disruptions and adjust flight schedules, minimizing delays and improving customer satisfaction.
How Big Data Analysis Works
Big data analysis works through steps to transform vast and varied data into valuable insights.
The process involves data collection, storage, processing, analysis, and visualization, enabling businesses to make data-driven decisions in real-time or near-real-time.
Here’s a breakdown of how big data analysis works:
1. Collecting Your Data
First, you gather data from all the different sources available to your business, like:
- Social media interactions
- Website analytics
- Transaction logs
- Sensor data if you’re in manufacturing or logistics
This data may be structured (like tables in databases), semi-structured (like emails or XML files), or completely unstructured (think videos or text files).
Tools such as Apache Kafka or cloud platforms help streamline the data collection process, ensuring you capture everything you need efficiently.
2. Storing and Managing Your Data
Once collected, you need somewhere to store your data in a way that allows for easy access and organization.
Here’s where data warehouses and data lakes come into play. Data lakes are ideal for storing raw or unstructured data, while data warehouses work better for structured data you frequently query.
Platforms like Amazon S3 and Snowflake are popular for setting up robust storage solutions. Storing your data well is essential, as it sets the foundation for future analysis.
3. Processing and Cleaning Your Data
Raw data often comes with imperfections—errors, duplicates, and inconsistencies—that can interfere with your analysis.
Here, you’ll clean, organize, and prepare the data, a step known as data wrangling. Using tools like Apache Spark or traditional ETL (Extract, Transform, Load) processes, you can structure your data effectively, ensuring that your analysis is based on reliable, high-quality information.
Think of this as tidying up your data so you can extract the most meaningful insights.
4. Analyzing Your Data
With your data prepared, you can dive into the analysis stage.
There are four main types of analytics that you can apply, each serving a different purpose:
- Descriptive Analytics: This type gives you a summary of what happened in the past. For example, analyzing sales data to see patterns in seasonal demand.
- Diagnostic Analytics: Here, you dig deeper to understand why something happened, like examining why a particular product saw a drop in sales.
- Predictive Analytics: Using historical data, you can forecast trends, such as predicting customer demand for a new product launch.
- Prescriptive Analytics: This is the most advanced form, suggesting the best action based on your data insights. For instance, data can be used to recommend which products to stock during a specific season.
Each type of analysis gives you a different perspective, allowing you to make well-rounded, data-driven decisions.
5. Visualizing and Reporting Your Insights
Once you’ve gathered your insights, the final step is making them accessible and understandable. This is where visualization tools like Tableau, Power BI, or Looker Studio become invaluable.
These tools turn complex data into visual representations—charts, graphs, and dashboards—that let you and your team grasp trends and results quickly.
Effective visualization helps ensure everyone in your organization can act on the insights, empowering you to make informed decisions faster.
How Big Data Analysis Helps Businesses Increase Their Revenue
Big data analysis helps businesses increase revenue by unlocking insights that drive smarter, more profitable decisions.
Here are some key ways big data analysis contributes to revenue growth:
1. Optimizing Pricing Strategies
With big data, you can analyze customer behavior, competitor prices, and market demand in real time, helping you set optimal prices.
Dynamic pricing, often used by e-commerce giants like Amazon, adjusts prices to reflect real-time demand and competitor actions, maximizing profitability.
2. Enhancing Marketing Campaigns
Big data enables targeted and personalized marketing, allowing you to engage customers with tailored offers.
By analyzing past campaigns and customer interactions, you can identify the content, timing, and channels that yield the highest conversions.
This targeted approach improves engagement and ROI, driving revenue from each campaign.
Businesses that implement personalized marketing strategies can achieve up to 8 times higher ROI compared to traditional methods.
3. Improving Customer Retention
Big data helps you understand customer preferences and predict behaviors, allowing you to address potential issues and tailor experiences that build loyalty proactively.
For instance, by analyzing feedback and purchase histories, companies can identify customers at risk of churning and target them with special offers or personalized support.
Research shows that improving customer retention by just 5% can lead to 25-95% revenue increases over time.
4. Streamlining Operations to Reduce Costs
Operational efficiency directly affects revenue growth by reducing unnecessary expenses and allocating resources more effectively.
Big data analysis identifies bottlenecks and inefficiencies in production or supply chains, enabling timely adjustments that optimize workflows.
For example, FedEx and UPS use big data to optimize delivery routes, reduce fuel costs, improve delivery times, and enhance customer satisfaction.
5. Driving Innovation and Product Development
Big data insights reveal unmet customer needs, helping you develop products and services that align closely with market demands.
Companies can innovate strategically by analyzing customer feedback, industry trends, and competitor offerings.
In industries like tech and consumer goods, big data insights drive new product features or entirely new product lines that meet evolving consumer expectations, boosting sales and market share.
6. Supporting Risk Management and Fraud Prevention
In sectors like finance and e-commerce, big data helps detect anomalies that could indicate fraud.
You can analyze transaction patterns and flag suspicious activities in real time, preventing revenue loss due to fraud.
Additionally, predictive analytics allows businesses to anticipate market risks, enabling proactive adjustments to protect revenue streams.
Challenges of Implementing Big Data Analysis
Implementing big data analysis offers significant advantages, but it also comes with challenges that businesses must address to realize its potential fully.
Here are some common obstacles:
1. Data Privacy and Security Concerns
With vast amounts of sensitive data collected, protecting it from unauthorized access and ensuring compliance with privacy regulations like GDPR is essential.
Businesses face challenges around securing data at every stage—collection, storage, and analysis—and failing to do so can lead to breaches, costly fines, and loss of customer trust.
Data security practices like encryption and access controls help, but managing security at scale can be complex and costly.
2. High Implementation and Maintenance Costs
Establishing a big data infrastructure involves significant upfront investment in technology, storage, and skilled personnel.
Cloud platforms and big data solutions can mitigate some costs, but ongoing maintenance, updates, and infrastructure scaling add to the financial burden.
Many small to mid-sized businesses may struggle to justify the high costs without a clear, immediate return on investment.
3. Data Quality and Integration Issues
Ensuring high-quality data is crucial for accurate insights, yet data from various sources may come in different formats and contain inconsistencies, duplicates, or errors.
This lack of uniformity makes it challenging to integrate data seamlessly.
Data cleansing processes are essential but time-intensive, requiring significant resources to ensure data accuracy and relevance.
4. Complexity in Data Management
Handling the "3Vs" of big data—volume, variety, and velocity—can be daunting.
Large volumes of data must be processed quickly and efficiently, especially in real-time applications.
Storage solutions like data lakes can help, but managing data complexity and ensuring smooth integration with existing systems adds another layer of difficulty.
Furthermore, legacy systems may not be compatible with new data technologies, requiring additional investment.
5. Resistance to Organizational Change
Implementing big data analysis often requires a cultural shift toward data-driven decision-making. Employees may resist changes in workflows or distrust data analytics, especially if they’re accustomed to intuition-based or traditional decision-making processes.
Encouraging organization-wide buy-in and providing training to help employees understand and embrace big data tools is crucial, but it takes time and consistent effort.
6. Skill Gap and Workforce Shortage
Another significant challenge in implementing big data analysis is the shortage of skilled professionals.
Data scientists, analysts, and engineers with expertise in big data technologies are in high demand, making it difficult for businesses to find and retain the right talent.
You can invest in training and product development programs to address this skill gap and upskill your existing workforce.
However, training can often be too expensive and challenging to implement effectively, especially when finding the right staff with the necessary expertise.
For this reason, IT staffing solutions better bridge the skill gap by providing access to specialized expertise on demand.
By augmenting your in-house team with external talent from partners like NeoWork, you can leverage the knowledge and experience of data professionals without the need for significant long-term investments.
Best Practices for Leveraging Big Data Analysis
To maximize the benefits of big data analysis and drive revenue growth, consider implementing these best practices:
1. Start with a Clear Business Objective
Before diving into big data analysis, clearly define your specific goals and key performance indicators (KPIs).
- What do you want to achieve?
- Is it increasing sales, improving customer retention, or optimizing operations?
Having a well-defined objective helps you focus your efforts and measure the success of your big data initiatives.
Identify the relevant data sources and metrics that align with your business goals. This may include customer data, sales data, website analytics, or social media insights.
Starting with a clear objective ensures that your big data analysis efforts are targeted and purposeful.
2. Invest in the Right Technology and Infrastructure
To effectively leverage big data analysis, invest in scalable and flexible big data platforms.
Look for solutions to handle your data's volume, variety, and velocity. Cloud-based platforms, such as Amazon Web Services (AWS) and Google Cloud Platform, offer scalable storage and processing capabilities, allowing you to handle growing data volumes easily.
Consider tools and technologies that enable real-time data processing and analysis, such as Apache Spark and Apache Kafka.
These technologies allow you to process and analyze data as it streams in, making timely decisions and responding to changing market conditions.
3. Foster a Data-Driven Culture
Cultivate a data-driven culture within your organization. Encourage data literacy and collaboration across departments.
Provide training and resources to help employees understand the value of data and how to use it effectively in their roles.
Break down data silos and promote data sharing across the organization. Establish data governance policies to ensure data quality, security, and privacy. By fostering a culture that values data-driven decision-making, you can unlock the full potential of big data analysis and drive innovation across your business.
4. Partner with Experienced Providers
Implementing big data analysis can be complex and resource-intensive.
So, partner with experienced big data providers who offer tailored solutions and guidance.
These providers have the expertise and tools to help you navigate big data challenges and accelerate your time-to-value.
You can also outsource big data projects to specialized providers, which allows you to access advanced big data analytics capabilities without significant in-house investments.
They can help you design and implement big data solutions that align with your business objectives and provide ongoing support and maintenance.
Why Leverage Big Data Analysis Through Outsourcing
Outsourcing big data analysis offers several strategic advantages, particularly for businesses looking to harness the power of big data without incurring the significant costs and complexities associated with in-house implementation.
Here’s why outsourcing is often a smart choice:
1. Access Specialized Expertise and Technologies
One of the primary benefits of outsourcing big data analysis is gaining access to domain-specific expertise that may not be available in-house.
Partners specializing in big data can help develop sophisticated algorithms, use advanced machine learning models, and implement powerful analytics tools to generate insights that drive business growth.
According to a study by McKinsey Global Institute, data-driven organizations are significantly more likely to outperform their peers in acquiring customers and achieving profitability, illustrating the transformative impact of big data analysis.
2. Scalable Solutions for Growing Data Needs
Big data outsourcing also offers scalability.
Whether analyzing customer data to optimize marketing strategies or using predictive analytics to improve operational efficiency, outsourcing ensures you can adapt as your business evolves.
3. Enhance Revenue Through Data-Driven Insights
Outsourced big data analysis can help businesses boost revenue by allowing them to craft highly targeted marketing campaigns, personalize customer interactions, and optimize pricing strategies.
By partnering with big data experts, you can uncover hidden trends, refine customer segmentation, and identify new growth opportunities.
Research shows that companies leveraging data-driven insights can experience a 5-6% increase in productivity and profitability.
4. Improve Operational Efficiency
Another significant advantage of outsourcing big data analysis is enhancing operational efficiency.
Big data analysis helps organizations streamline their processes by identifying inefficiencies, bottlenecks, and areas for improvement.
For example, predictive maintenance models can forecast equipment failures and help plan proactive maintenance schedules, reducing downtime and repair costs.
5. Long-Term Strategic Advantage
Outsourcing big data analysis positions businesses for long-term success.
As data continues to grow exponentially, the ability to extract valuable insights will increasingly become a competitive differentiator.
Leveraging the expertise of outsourced providers will help you stay ahead of market trends.
Partner with NeoWork for World-Class Talent
Outsourcing is one of the best ways to leverage big data analysis for your business, as it brings access to:
- Specialized expertise
- Cost-effective solutions
- Scalable resources without the complexities of an in-house setup
Big data analysis also requires advanced skills in data science, machine learning, statistical analysis, and the right infrastructure and tools to manage, process, and derive insights from vast datasets.
Outsourcing this function allows you to tap into top-notch capabilities and focus your internal resources on core business activities.
Partnering with firms like NeoWork provides access to world-class talent, handpicked to match high standards, with only 3.2% of candidates making it through their rigorous recruitment process.
NeoWork supports businesses in areas directly beneficial to data-driven operations, such as AI training and MVP (Minimum Viable Product) development, customer experience optimization, and technical support—all essential for effectively handling and leveraging data insights.
NeoWork's team members work as an extension of your business, integrating seamlessly with your internal operations to provide round-the-clock support, workforce management, and quality assurance, ensuring that big data processes align with your business objectives and performance standards.
Reach out for a free consultation and see how NeoWork’s elite talent can streamline your operations and optimize your data strategy.
How Big Data Analysis Helps Businesses Increase Their Revenue
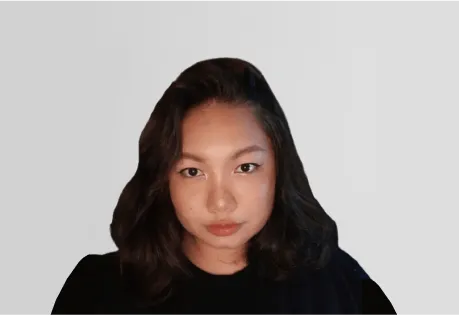
Have you ever wondered what separates thriving businesses from those that struggle to grow?
How do some companies consistently make smarter decisions, predict customer needs, and increase revenue—while others seem to miss the mark? The answer often lies in one powerful tool: big data analysis.
Imagine being able to foresee market trends, optimize your pricing, or pinpoint exactly what your customers want.
But the real question is: how can your business leverage this data to increase profits? In this article, we’ll explore how big data analysis provides a competitive edge to help your company boost its revenue.
What is Big Data Analysis?
Big data analysis examines large, complex datasets to uncover hidden patterns, correlations, and other valuable insights.
It involves using advanced analytics techniques, such as machine learning, data mining, and predictive modeling, to make sense of the data and extract actionable intelligence.
Big data analysis aims to help businesses:
- Make data-driven decisions
- Optimize processes
- Identify new opportunities for growth
By leveraging the power of big data, companies can gain a deeper understanding of their customers, market trends, and operational performance.
Examples of Big Data Analysis in Business
Big data analysis has applications across various business functions and industries. Here are some examples:
- Analyzing customer behavior and preferences: By examining data from social media, website interactions, and purchase history, businesses can gain insights into customer preferences, segment their audience, and personalize marketing efforts.
- Optimizing supply chain and logistics: Big data analysis can help companies streamline their supply chain by identifying inefficiencies, predicting demand, and optimizing inventory management.
- Detecting and preventing fraud: Financial institutions and insurance companies use big data analysis to identify suspicious patterns and prevent fraudulent activities.
- Improving marketing campaigns and personalization: By analyzing customer data, businesses can create targeted marketing campaigns, recommend relevant products or services, and enhance the overall customer experience.
Benefits of Big Data Analysis for Businesses
Now that you understand what big data analysis is and how it works, let's explore the tangible benefits it offers to businesses.
1. Increased Revenue
One of the primary advantages of big data analysis is its potential to drive revenue growth.
By examining vast amounts of data, you can uncover new business opportunities and revenue streams that may have otherwise gone unnoticed.
For example, organizations that leverage big data report an average 8% increase in revenues and a 10% reduction in expenses.
Big data analysis also enables you to optimize pricing and promotions. You can adjust real-time prices to maximize profitability by understanding customer behavior and market trends.
Additionally, analyzing the effectiveness of past promotions can help you create more targeted and successful campaigns in the future.
2. Improved Customer Experience
Big data analysis empowers you to personalize products, services, and marketing efforts based on customer preferences and behaviors. In fact, 76% of consumers are more likely to choose a brand that offers personalized experiences.
By leveraging data from various touchpoints, such as website interactions, social media, and customer support channels, you can gain a 360-degree view of your customers and tailor your offerings accordingly.
Moreover, big data analysis can help you predict and prevent customer churn.
By identifying patterns and risk factors associated with customer attrition, you can proactively reach out to at-risk customers and offer personalized incentives to retain their business.
3. Enhanced Operational Efficiency
Big data analysis significantly improves operational efficiency by streamlining processes and reducing costs.
For instance, companies utilizing big data through AI-enabled supply-chain management can improve logistic costs by up to 15%.
For example, predictive maintenance powered by big data reduces equipment downtime in manufacturing.
Manufacturers prevent unexpected breakdowns by analyzing machine data and predicting when maintenance will be required, saving time and money.
General Electric, for example, uses big data to monitor its engines, ensuring they run optimally, saving millions in maintenance costs.
4. Better Decision Making
Big data analysis empowers you to make informed, data-driven decisions based on real-time insights.
Approximately 69% of organizations report that big data initiatives lead to better strategic decision-making, enabling them to respond more effectively to market changes.
You can comprehensively understand your business performance, customer behavior, and market trends by analyzing vast amounts of data from various sources. This enables you to make strategic decisions quickly and confidently rather than relying on intuition or limited information.
Furthermore, big data analysis helps you identify and mitigate potential risks. By detecting anomalies, fraud, or other threats early on, you can take proactive measures to protect your business and minimize the impact of adverse events.
5. Risk Management and Fraud Detection
Businesses can detect anomalies and flag potential fraud in real time by continuously analyzing transactions, especially in sectors like finance and e-commerce.
For example, banks use big data to monitor transactions and identify patterns indicative of credit card fraud.
This proactive risk management approach saves the institution and its customers from financial losses.
Risk management also includes anticipating operational and market risks, allowing companies to create contingency plans.
For example, airlines use big data to predict weather disruptions and adjust flight schedules, minimizing delays and improving customer satisfaction.
How Big Data Analysis Works
Big data analysis works through steps to transform vast and varied data into valuable insights.
The process involves data collection, storage, processing, analysis, and visualization, enabling businesses to make data-driven decisions in real-time or near-real-time.
Here’s a breakdown of how big data analysis works:
1. Collecting Your Data
First, you gather data from all the different sources available to your business, like:
- Social media interactions
- Website analytics
- Transaction logs
- Sensor data if you’re in manufacturing or logistics
This data may be structured (like tables in databases), semi-structured (like emails or XML files), or completely unstructured (think videos or text files).
Tools such as Apache Kafka or cloud platforms help streamline the data collection process, ensuring you capture everything you need efficiently.
2. Storing and Managing Your Data
Once collected, you need somewhere to store your data in a way that allows for easy access and organization.
Here’s where data warehouses and data lakes come into play. Data lakes are ideal for storing raw or unstructured data, while data warehouses work better for structured data you frequently query.
Platforms like Amazon S3 and Snowflake are popular for setting up robust storage solutions. Storing your data well is essential, as it sets the foundation for future analysis.
3. Processing and Cleaning Your Data
Raw data often comes with imperfections—errors, duplicates, and inconsistencies—that can interfere with your analysis.
Here, you’ll clean, organize, and prepare the data, a step known as data wrangling. Using tools like Apache Spark or traditional ETL (Extract, Transform, Load) processes, you can structure your data effectively, ensuring that your analysis is based on reliable, high-quality information.
Think of this as tidying up your data so you can extract the most meaningful insights.
4. Analyzing Your Data
With your data prepared, you can dive into the analysis stage.
There are four main types of analytics that you can apply, each serving a different purpose:
- Descriptive Analytics: This type gives you a summary of what happened in the past. For example, analyzing sales data to see patterns in seasonal demand.
- Diagnostic Analytics: Here, you dig deeper to understand why something happened, like examining why a particular product saw a drop in sales.
- Predictive Analytics: Using historical data, you can forecast trends, such as predicting customer demand for a new product launch.
- Prescriptive Analytics: This is the most advanced form, suggesting the best action based on your data insights. For instance, data can be used to recommend which products to stock during a specific season.
Each type of analysis gives you a different perspective, allowing you to make well-rounded, data-driven decisions.
5. Visualizing and Reporting Your Insights
Once you’ve gathered your insights, the final step is making them accessible and understandable. This is where visualization tools like Tableau, Power BI, or Looker Studio become invaluable.
These tools turn complex data into visual representations—charts, graphs, and dashboards—that let you and your team grasp trends and results quickly.
Effective visualization helps ensure everyone in your organization can act on the insights, empowering you to make informed decisions faster.
How Big Data Analysis Helps Businesses Increase Their Revenue
Big data analysis helps businesses increase revenue by unlocking insights that drive smarter, more profitable decisions.
Here are some key ways big data analysis contributes to revenue growth:
1. Optimizing Pricing Strategies
With big data, you can analyze customer behavior, competitor prices, and market demand in real time, helping you set optimal prices.
Dynamic pricing, often used by e-commerce giants like Amazon, adjusts prices to reflect real-time demand and competitor actions, maximizing profitability.
2. Enhancing Marketing Campaigns
Big data enables targeted and personalized marketing, allowing you to engage customers with tailored offers.
By analyzing past campaigns and customer interactions, you can identify the content, timing, and channels that yield the highest conversions.
This targeted approach improves engagement and ROI, driving revenue from each campaign.
Businesses that implement personalized marketing strategies can achieve up to 8 times higher ROI compared to traditional methods.
3. Improving Customer Retention
Big data helps you understand customer preferences and predict behaviors, allowing you to address potential issues and tailor experiences that build loyalty proactively.
For instance, by analyzing feedback and purchase histories, companies can identify customers at risk of churning and target them with special offers or personalized support.
Research shows that improving customer retention by just 5% can lead to 25-95% revenue increases over time.
4. Streamlining Operations to Reduce Costs
Operational efficiency directly affects revenue growth by reducing unnecessary expenses and allocating resources more effectively.
Big data analysis identifies bottlenecks and inefficiencies in production or supply chains, enabling timely adjustments that optimize workflows.
For example, FedEx and UPS use big data to optimize delivery routes, reduce fuel costs, improve delivery times, and enhance customer satisfaction.
5. Driving Innovation and Product Development
Big data insights reveal unmet customer needs, helping you develop products and services that align closely with market demands.
Companies can innovate strategically by analyzing customer feedback, industry trends, and competitor offerings.
In industries like tech and consumer goods, big data insights drive new product features or entirely new product lines that meet evolving consumer expectations, boosting sales and market share.
6. Supporting Risk Management and Fraud Prevention
In sectors like finance and e-commerce, big data helps detect anomalies that could indicate fraud.
You can analyze transaction patterns and flag suspicious activities in real time, preventing revenue loss due to fraud.
Additionally, predictive analytics allows businesses to anticipate market risks, enabling proactive adjustments to protect revenue streams.
Challenges of Implementing Big Data Analysis
Implementing big data analysis offers significant advantages, but it also comes with challenges that businesses must address to realize its potential fully.
Here are some common obstacles:
1. Data Privacy and Security Concerns
With vast amounts of sensitive data collected, protecting it from unauthorized access and ensuring compliance with privacy regulations like GDPR is essential.
Businesses face challenges around securing data at every stage—collection, storage, and analysis—and failing to do so can lead to breaches, costly fines, and loss of customer trust.
Data security practices like encryption and access controls help, but managing security at scale can be complex and costly.
2. High Implementation and Maintenance Costs
Establishing a big data infrastructure involves significant upfront investment in technology, storage, and skilled personnel.
Cloud platforms and big data solutions can mitigate some costs, but ongoing maintenance, updates, and infrastructure scaling add to the financial burden.
Many small to mid-sized businesses may struggle to justify the high costs without a clear, immediate return on investment.
3. Data Quality and Integration Issues
Ensuring high-quality data is crucial for accurate insights, yet data from various sources may come in different formats and contain inconsistencies, duplicates, or errors.
This lack of uniformity makes it challenging to integrate data seamlessly.
Data cleansing processes are essential but time-intensive, requiring significant resources to ensure data accuracy and relevance.
4. Complexity in Data Management
Handling the "3Vs" of big data—volume, variety, and velocity—can be daunting.
Large volumes of data must be processed quickly and efficiently, especially in real-time applications.
Storage solutions like data lakes can help, but managing data complexity and ensuring smooth integration with existing systems adds another layer of difficulty.
Furthermore, legacy systems may not be compatible with new data technologies, requiring additional investment.
5. Resistance to Organizational Change
Implementing big data analysis often requires a cultural shift toward data-driven decision-making. Employees may resist changes in workflows or distrust data analytics, especially if they’re accustomed to intuition-based or traditional decision-making processes.
Encouraging organization-wide buy-in and providing training to help employees understand and embrace big data tools is crucial, but it takes time and consistent effort.
6. Skill Gap and Workforce Shortage
Another significant challenge in implementing big data analysis is the shortage of skilled professionals.
Data scientists, analysts, and engineers with expertise in big data technologies are in high demand, making it difficult for businesses to find and retain the right talent.
You can invest in training and product development programs to address this skill gap and upskill your existing workforce.
However, training can often be too expensive and challenging to implement effectively, especially when finding the right staff with the necessary expertise.
For this reason, IT staffing solutions better bridge the skill gap by providing access to specialized expertise on demand.
By augmenting your in-house team with external talent from partners like NeoWork, you can leverage the knowledge and experience of data professionals without the need for significant long-term investments.
Best Practices for Leveraging Big Data Analysis
To maximize the benefits of big data analysis and drive revenue growth, consider implementing these best practices:
1. Start with a Clear Business Objective
Before diving into big data analysis, clearly define your specific goals and key performance indicators (KPIs).
- What do you want to achieve?
- Is it increasing sales, improving customer retention, or optimizing operations?
Having a well-defined objective helps you focus your efforts and measure the success of your big data initiatives.
Identify the relevant data sources and metrics that align with your business goals. This may include customer data, sales data, website analytics, or social media insights.
Starting with a clear objective ensures that your big data analysis efforts are targeted and purposeful.
2. Invest in the Right Technology and Infrastructure
To effectively leverage big data analysis, invest in scalable and flexible big data platforms.
Look for solutions to handle your data's volume, variety, and velocity. Cloud-based platforms, such as Amazon Web Services (AWS) and Google Cloud Platform, offer scalable storage and processing capabilities, allowing you to handle growing data volumes easily.
Consider tools and technologies that enable real-time data processing and analysis, such as Apache Spark and Apache Kafka.
These technologies allow you to process and analyze data as it streams in, making timely decisions and responding to changing market conditions.
3. Foster a Data-Driven Culture
Cultivate a data-driven culture within your organization. Encourage data literacy and collaboration across departments.
Provide training and resources to help employees understand the value of data and how to use it effectively in their roles.
Break down data silos and promote data sharing across the organization. Establish data governance policies to ensure data quality, security, and privacy. By fostering a culture that values data-driven decision-making, you can unlock the full potential of big data analysis and drive innovation across your business.
4. Partner with Experienced Providers
Implementing big data analysis can be complex and resource-intensive.
So, partner with experienced big data providers who offer tailored solutions and guidance.
These providers have the expertise and tools to help you navigate big data challenges and accelerate your time-to-value.
You can also outsource big data projects to specialized providers, which allows you to access advanced big data analytics capabilities without significant in-house investments.
They can help you design and implement big data solutions that align with your business objectives and provide ongoing support and maintenance.
Why Leverage Big Data Analysis Through Outsourcing
Outsourcing big data analysis offers several strategic advantages, particularly for businesses looking to harness the power of big data without incurring the significant costs and complexities associated with in-house implementation.
Here’s why outsourcing is often a smart choice:
1. Access Specialized Expertise and Technologies
One of the primary benefits of outsourcing big data analysis is gaining access to domain-specific expertise that may not be available in-house.
Partners specializing in big data can help develop sophisticated algorithms, use advanced machine learning models, and implement powerful analytics tools to generate insights that drive business growth.
According to a study by McKinsey Global Institute, data-driven organizations are significantly more likely to outperform their peers in acquiring customers and achieving profitability, illustrating the transformative impact of big data analysis.
2. Scalable Solutions for Growing Data Needs
Big data outsourcing also offers scalability.
Whether analyzing customer data to optimize marketing strategies or using predictive analytics to improve operational efficiency, outsourcing ensures you can adapt as your business evolves.
3. Enhance Revenue Through Data-Driven Insights
Outsourced big data analysis can help businesses boost revenue by allowing them to craft highly targeted marketing campaigns, personalize customer interactions, and optimize pricing strategies.
By partnering with big data experts, you can uncover hidden trends, refine customer segmentation, and identify new growth opportunities.
Research shows that companies leveraging data-driven insights can experience a 5-6% increase in productivity and profitability.
4. Improve Operational Efficiency
Another significant advantage of outsourcing big data analysis is enhancing operational efficiency.
Big data analysis helps organizations streamline their processes by identifying inefficiencies, bottlenecks, and areas for improvement.
For example, predictive maintenance models can forecast equipment failures and help plan proactive maintenance schedules, reducing downtime and repair costs.
5. Long-Term Strategic Advantage
Outsourcing big data analysis positions businesses for long-term success.
As data continues to grow exponentially, the ability to extract valuable insights will increasingly become a competitive differentiator.
Leveraging the expertise of outsourced providers will help you stay ahead of market trends.
Partner with NeoWork for World-Class Talent
Outsourcing is one of the best ways to leverage big data analysis for your business, as it brings access to:
- Specialized expertise
- Cost-effective solutions
- Scalable resources without the complexities of an in-house setup
Big data analysis also requires advanced skills in data science, machine learning, statistical analysis, and the right infrastructure and tools to manage, process, and derive insights from vast datasets.
Outsourcing this function allows you to tap into top-notch capabilities and focus your internal resources on core business activities.
Partnering with firms like NeoWork provides access to world-class talent, handpicked to match high standards, with only 3.2% of candidates making it through their rigorous recruitment process.
NeoWork supports businesses in areas directly beneficial to data-driven operations, such as AI training and MVP (Minimum Viable Product) development, customer experience optimization, and technical support—all essential for effectively handling and leveraging data insights.
NeoWork's team members work as an extension of your business, integrating seamlessly with your internal operations to provide round-the-clock support, workforce management, and quality assurance, ensuring that big data processes align with your business objectives and performance standards.
Reach out for a free consultation and see how NeoWork’s elite talent can streamline your operations and optimize your data strategy.
Topics
